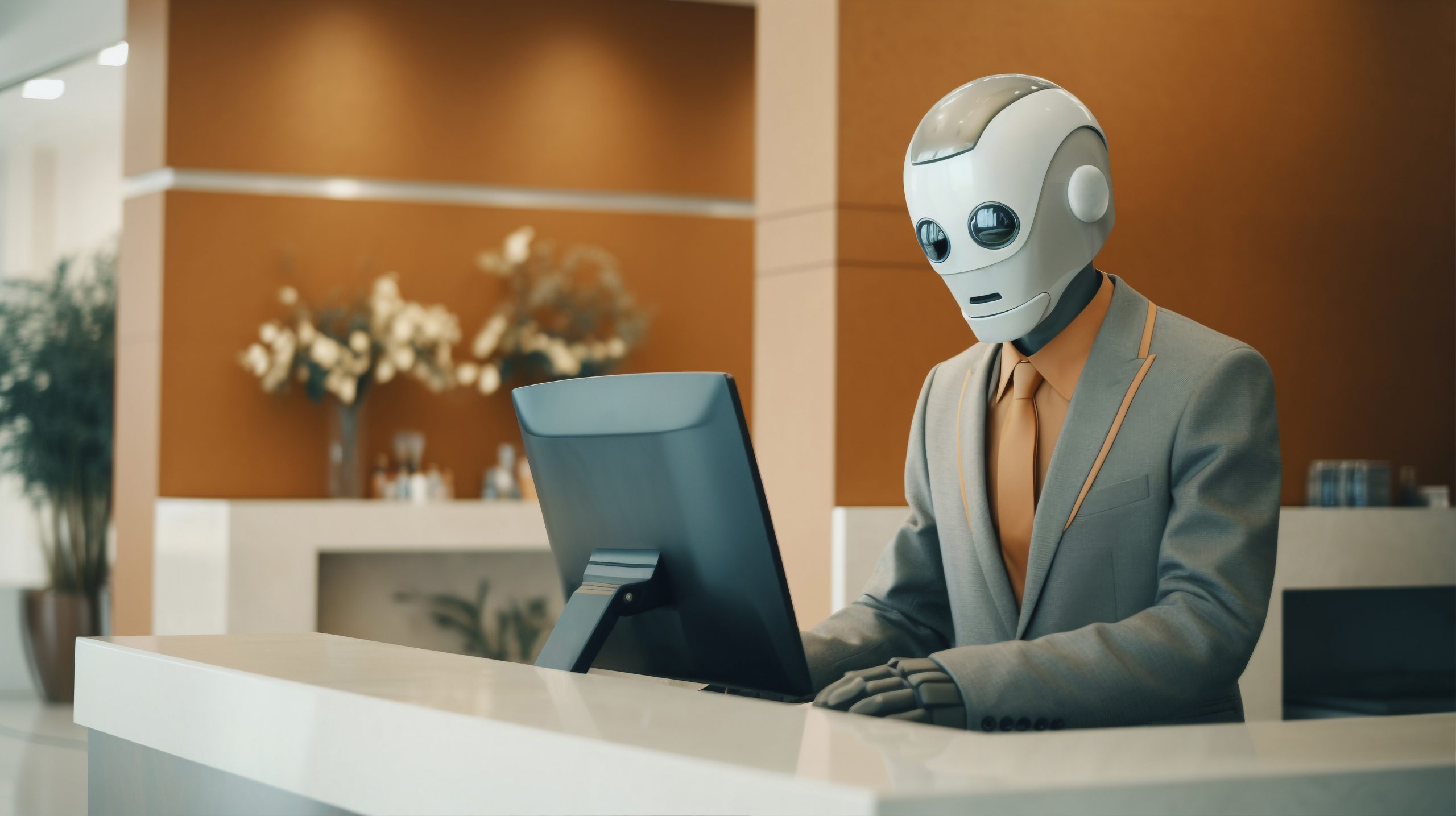
Artificial Intelligence (AI) has been a game-changer in many fields, from medicine to finance and marketing. One of the most intriguing applications of AI is Generative AI, which allows machines to create new content. Generative AI can be used for a wide variety of tasks, from generating realistic images and videos to creating natural language texts and even music.
In this blog, we’ll delve deep into the world of generative artificial intelligence (AI), unravelling its intricate mechanisms, awe-inspiring capabilities, and the groundbreaking innovations it brings forth. Join us as we uncover the nitty-gritty of generative AI, igniting your curiosity and expanding your horizons in the ever-evolving landscape of technological wonders. Let’s dive in and discover the fascinating tech behind generative AI.
Generative Artificial Intelligence (AI) refers to a type of AI that is capable of creating new content or data, such as images, text, or music, that is similar to human-created content. Generative AI uses machine learning algorithms to analyse and learn from existing data sets and then generate new content that is similar in style and structure to the original data.
Generative AI is an exciting type of technology that allows for the creation of various types of content, including text, imagery, audio, and synthetic data. With new user interfaces, it is now easier than ever to create high-quality text, graphics, and videos in mere seconds.
While chatbots introduced generative AI in the 1960s, it was the introduction of generative adversarial networks, or GANs, in 2014 that allowed for convincingly authentic images, videos, and audio of real people.
Recent advancements in large language models and multimodal AI have taken generative AI to a new level, allowing it to write engaging text, paint photorealistic images, and even create sitcoms in real time. Despite early issues with accuracy, bias, and hallucinations, the potential of generative AI to transform business processes through code-writing, drug development, product design, and supply chain transformation is immense.
Generative AI has many benefits, but it also comes with some major downsides from misuse – deep fakes and cybersecurity attacks being two growing concerns. That said, recent breakthroughs in generative transformers and attention models have made it possible to train ever-larger models on billions of text pages, resulting in deeper answers and the ability to track connections between words across pages and genres.
All in all, Generative AI has the potential to revolutionise the way we create and consume content, ushering in a new era of productivity, creativity, and digital innovation.
So, what are the differences between Generative AI and Traditional AI? Generative AI is all about producing new content, while Traditional AI is geared towards detecting patterns and making decisions. Generative AI uses cutting-edge neural network techniques such as transformers, GANs, and VAEs. Conversely, Traditional AI techniques include convolutional neural networks, recurrent neural networks, and reinforcement learning.
Furthermore, with Generative AI, you can get started by submitting a prompt or data set to guide content generation and explore variations through an iterative process. Traditional AI algorithms, on the other hand, rely on newer data sets to deliver a straightforward and optimal result.
Now that we’ve distinguished generative AI technology from its parent technology let’s have a quick look at what could be on the horizon for this modern innovation. ChatGPT has demonstrated incredible depth and ease, promising the widespread adoption of generative AI. Despite initial implementation challenges, tools are being developed to detect AI-generated text, images, and video, and to track the origin of information, creating more trustworthy AI.
As AI development platforms improve and evolve, generative AI capabilities will follow suit and further the creation of text, images, video, 3D content, drugs, supply chains, logistics, and business processes. One-off tools like ChatGPT are beneficial, but embedding generative AI capabilities directly into existing tools will have the most significant impact.
Grammar checkers, design tools, and training tools will become more advanced, providing practical recommendations, promoting efficient training, and transforming workflows. Considering how big the impact has already been, Generative AI is sure to revolutionise the way we work in many exciting ways.
As an example, you can check out Runway’s Gen-1 AI in the video below, demonstrating the next step forward for generative AI.
At its core, generative AI relies on deep learning models that are trained on large datasets of examples. These models learn to recognise patterns and structures in the data and can then generate new data that resembles the original. For instance, in the case of natural language generation, the models learn to generate text that is similar in style and content to a given corpus of text.
Unlike traditional computing models that are pre-programmed, generative AI uses a vast amount of data to learn how to create new content that is similar or unique to the input it receives. Generative AI’s ability to create something novel has attracted the attention of many researchers, and there are still many possibilities yet to be explored.
It all begins with a prompt, which can take the form of text, images, videos, designs, or musical notes – any input that the AI system can process. The AI algorithms then use this input to produce new content, which can range from written essays, and problem-solving solutions, all the way to lifelike fakes created from images or audio of people.
Earlier iterations of generative AI required users to submit data via complex APIs and specialised tools and programming languages such as Python. Nowadays, developers of generative AI technology are improving user experience by allowing for plain language requests. In addition, users are given the ability to customise the results by giving feedback on the style, tone, and other elements they desire the generated content to reflect.
The process of generating content using a generative AI model typically starts with the effective encoding of the desired representation. For instance, the case of a text-based generative AI model involves finding a method to represent words as vectors that capture the similarity between frequently co-occurring words or words with similar meanings within sentences.
Significant advancements in Language and Learning Models (LLMs) research have enabled the industry to extend this process to various other domains. Now, generative AI models can effectively represent patterns found in images, sounds, proteins, DNA sequences, drugs, and even 3D designs. This approach facilitates an efficient representation of the desired content type, allowing for generating valuable and diverse variations through iterative processes.
Generative AI models use various algorithms to process and represent content. For instance, natural language processing techniques manipulate raw characters into sentences, parts of speech, entities, and actions encoded as vectors. Similarly, visual elements represent images in vector form. However, these techniques can also encode biases and other negative elements from the training data.
Developers apply a neural network that matches their world representation to generate new content in response to a query. Examples of neural networks that generate realistic content include Generative Adversarial Networks (GANs) and Variational Auto Encoders (VAEs). Transformers like Google’s BERT and OpenAI’s GPT, and AlphaFold can also encode and generate language, images, and other types of content.
Neural networks, the underlying framework of numerous AI and machine learning applications prevalent today, revolutionised the approach. They sought to emulate the intricate workings of the human brain, enabling these networks to acquire knowledge by discerning patterns within existing datasets.
In their nascent stages during the 1950s and 1960s, neural networks faced constraints due to insufficient computational capabilities and limited data availability. However, the landscape shifted dramatically in the mid-2000s with the emergence of big data and advancements in computer hardware, propelling neural networks towards their practical implementation in content generation.
AI has been generating content programmatically for decades. Neural networks revolutionised generative AI by learning rules from existing data sets. Advances in computer hardware in the mid-2000s paved the way for neural networks to become widely adopted. Furthermore, new techniques like generative adversarial networks and transformers make remarkable AI-generated content possible today.
To effectively deploy a generative AI model, it is crucial to train it for a specific use case scenario. Recent advancements in Language and Learning Models (LLMs) have greatly facilitated this process, offering a solid foundation for customising applications across different domains. One notable example is the widely known GPT model developed by OpenAI, which has demonstrated its versatility in tasks such as text generation, code generation, and image synthesis based on textual descriptions.
Training a generative AI model involves the fine-tuning of its parameters according to the desired use case, followed by further refinement using specific training data. For instance, a call centre may train a chatbot using datasets comprising typical customer queries and corresponding responses from service agents. This training enables the chatbot to address various customer needs effectively.
On the other hand, an image generation application, unlike text-based training, may start with labelled data describing desired image content and style. This approach trains the model to generate new and relevant images based on the provided labels.
By tailoring the model’s parameters and fine-tuning it with appropriate training data, generative AI models can be customised to excel in specific use cases, allowing them to generate high-quality outputs aligned with the intended application.
One of the most popular types of generative AI for natural language generation is known as a language model. Language models are trained on large amounts of text data, such as books or articles, and can generate new text based on the patterns they have learned. There are several types of language models, but the most common is based on recurrent neural networks (RNNs) or transformers.
RNN-based language models work by processing the input text one word at a time and using the previous words to predict the next word. This allows the model to generate text that flows naturally and makes sense in context. However, RNNs can struggle with longer texts or more complex structures, which is where transformer-based models come in.
Transformer-based models, such as OpenAI’s GPT (Generative Pre-trained Transformer) series, use a different approach to language generation. Instead of processing the input text sequentially, they use attention mechanisms to weigh the importance of each word in the text and generate new text based on those weights. This allows them to handle longer texts and more complex structures than RNN-based models.
Generative AI holds vast potential across various business domains, facilitating the interpretation, comprehension, and automatic generation of content. Developers are actively exploring ways to leverage generative AI in order to enhance existing workflows and even transform them entirely to harness the technology’s capabilities. The integration of generative AI can yield numerous benefits, including those listed below:
Essentially, by incorporating generative AI, businesses can unlock these advantages, driving productivity and efficiency to new heights.
The initial applications of generative AI have revealed numerous inherent limitations that must be addressed. Many of these arise from the specific methodologies employed to implement various use cases. For instance, while a summarised version of a complex topic may be more readable, it lacks the ability for users to verify the origins of the information as it omits the supporting sources.
Consider the following limitations when implementing or utilising a generative AI application.
Considering everything beneficial that can be done, addressing these limitations is crucial to ensure the responsible and effective implementation of generative AI technologies.
The rapid advancement and adoption of generative AI have also sparked a range of other concerns, encompassing the quality of outcomes, the potential for misuse, and the disruption of established business models.
While generative AI has enormous potential for creativity and innovation, it raises important ethical and social questions. As with any new technology, it is important to consider the ethical implications and use it responsibly. But, in its current state, generative AI presents several specific issues that warrant attention. These include:
This concern has been propping up everywhere since the emergence of generative AI tools. But how valid is it? Is it killing our creativity and imagination, or is it another tool in our toolbox? It’s a phenomenal tool; we’ll give it that!
The impact of generative AI on human productivity and laziness is a complex and emerging topic with varying perspectives. While generative AI technologies have undoubtedly automated certain tasks and made certain tasks more efficient and easier to accomplish, it’s not entirely accurate to say that they are solely responsible for making us lazy. Instead, they can be seen as tools that can either enhance or diminish our productivity, depending on how we choose to use them.
One of the biggest advantages of Generative AI is that it can automate repetitive or mundane tasks, freeing up human time and energy to focus on more creative and complex initiatives This can lead to increased productivity, innovation, and the ability to tackle more challenging problems. For example, AI-powered tools can automate data analysis, image generation, or content creation, allowing professionals to devote their attention to higher-level decision-making and problem-solving.
However, on the flip side, if individuals become overly reliant on AI and neglect developing their own skills or critical thinking abilities, it could potentially lead to a sense of complacency and, in fact, laziness. Therefore, It’s important for individuals to strike a balance between leveraging AI technologies and actively engaging in personal growth and development. Use it to augment your capabilities, not replace them.
Another major concern on everyone’s lips, can generative AI ultimately lead some jobs to obsolescence. While it’s true that generative AI can do some of the work humans are doing in a better way, it doesn’t necessarily mean that it will eventually replace humans as a consequence.
On the contrary, we need to look at it as a productivity-enhancing technology facilitating focus on high-value activities as opposed to a job-killer. In fact, Generative AI is more likely to replace specific tasks rather than entire jobs, and this can be seen as a positive development given the current state of productivity and wage growth. It is important not to dismiss the potential benefits of generative AI prematurely.
According to our estimates, around half a million Australians work in occupations that are most exposed to large-language models like ChatGPT, Bing, or Bard. This diverse group includes professionals such as telemarketers, educators, consultants, psychologists, counsellors, judges, and lawyers.
Fortunately, there is enough evidence suggesting that generative AI will enhance jobs rather than entirely replace them. For the top 10 most exposed occupations, generative AI affects only about one-third of the advertised tasks associated with each job, on average.
Historically, this pattern is not surprising. While automation is often assumed to eliminate jobs, it rarely does so. Research by Boston University economist James Bessen reveals that of the 270 occupations listed in the US census in 1950, only one no longer exists due to automation—the elevator operator.
Technology does not eliminate jobs; it transforms them. When properly harnessed, technology can create new jobs. Very soon, we can expect to see job ads for roles such as AI governance executives, cyber-physical security analysts, and predictive maintenance engineers. If tools like ChatGPT replace certain tasks in our work, it means we can redirect our time, energy, and expertise towards higher-skilled and higher-value tasks. This can lead to increased productivity, better wages, and more engaging and meaningful work that’s uniquely human.
Studies have shown that businesses implementing AI for customer support experience reduced response times by 40% and increased customer satisfaction by 35%. These improvements in efficiency translate into improved productivity as employees focus on higher-value tasks that contribute more to organisational growth.
To summarise, contrary to popular belief, the rise of artificial intelligence does not necessarily mean a doomsday scenario. At the same time, it’s not an assured ticket to a perfect world. The magnitude of change with AI is substantial but manageable with responsible development and implementation. Let’s not extinguish its potential before it proves its value for a prosperous future.
One of the most important applications of generative AI is in the field of creative industries, such as advertising, art, and marketing. For example, generative AI can be used to create new designs for advertisements or generate new pieces of art or content that are similar in style to those created by human artists. This can help to save time and resources, as well as to expand the range of creative output that is possible.
Emerging generative AI technologies have been likened to previous general-purpose technologies such as steam power, electricity, and computing, as they have the potential to impact diverse industries and use cases significantly. However, it is important to recognise that, similar to previous technological advancements, it may take a considerable time for optimal workflows to be developed and fully leverage the potential of generative AI rather than merely accelerating specific segments of existing processes.
All that said, here are several other ways in which generative AI applications could influence various ranges of industries.
The integration of generative AI across these industries has the potential to revolutionise workflows, improve efficiency, and unlock new creative possibilities.
Generative AI has come a long way in recent years, and we now have some impressive real-life examples to showcase its potential.
Midjourney exemplifies the potential of generative AI in transforming natural language prompts into captivating images. It stands among the prominent players in the AI landscape, alongside notable counterparts like DALL-E and Stable Diffusion, all leveraging machine learning for image generation.
Using Midjourney, you can effortlessly create high-quality images by simply providing text-based prompts. The convenience lies in its accessibility, as it operates seamlessly within the Discord chat application, eliminating the need for specialised hardware or software. However, it’s important to note that a subscription to a Midjourney plan is required to access its image generation capabilities, unlike some competitors who offer a limited number of free image generations.
Nonetheless, the barrier to entry with Midjourney remains remarkably low, enabling anyone to generate realistic images swiftly within minutes. The outcomes span a spectrum, ranging from eerily accurate representations to visually stunning compositions, solely dependent upon the nature of the prompt provided.
OpenAI’s ChatGPT is another generative AI-driven tool that generates human-like responses through text-based prompts and conversations. ChatGPT is a great example of generative AI, which demonstrates the incredible potential of generative AI to create content that is both realistic and useful.
In November 2022, the world witnessed the meteoric rise of this AI-powered chatbot, which was built using OpenAI’s GPT-3.5 implementation. OpenAI introduced this novel approach to engaging and refining text responses through a chat interface incorporating interactive feedback. This marked a departure from earlier versions of GPT, which were solely accessible via an API. Subsequently, GPT-4 was unveiled on March 14, 2023, bringing further advancements in AI language models.
ChatGPT changes robotic conversational experiences as we know them by incorporating the context and history of its user interactions, simulating authentic conversations. This feature enhanced the chatbot’s ability to provide contextually relevant and coherent responses.
The introduction of the new GPT interface has garnered immense popularity, capturing the attention of industry giants. Microsoft, recognising its potential, made a substantial investment in OpenAI and integrated a version of GPT into its Bing search engine, aiming to enhance search experiences and deliver more comprehensive and accurate information to users.
The collaboration between OpenAI and Microsoft signifies the growing recognition and adoption of advanced AI language models, paving the way for further advancements in natural language processing and chatbot technologies.
Google was at the forefront of transformer AI techniques initially, where it comprehended and processed various forms of content. The company was kind enough to distribute some of these models to researchers as open-source. However, it never provided a public means for these models.
As Microsoft integrated GPT into Bing, it prompted Google to hurry and launch a public-facing chatbot, the Google Bard. Unfortunately, the chatbot’s flawed debut impacted Google’s stock price negatively. It infamously mistakenly cited the discovery of a planet in a foreign solar system by the Webb telescope as the first one.
On the other hand, Microsoft and ChatGPT implementations were also plagued by precision failures and unstable performance in their early days. So, it’s best to watch this space as the search giants slowly figure out a way to incorporate generative AI into their algorithms.
MusicLM is another groundbreaking new technology that utilises generative AI to create remarkable musical compositions. This innovative platform is designed to mimic the style, rhythm, and tone of real-life instruments to produce unique and compelling melodies.
In contrast to traditional music-making software that requires human input, MusicLM uses algorithms to generate original songs and tracks based on specific parameters and inputs. By combining cutting-edge machine learning algorithms with sophisticated music composition tools, MusicLM unlocks creative possibilities that were once thought impossible.
With MusicLM, anyone can create breathtaking music with just a few clicks of a button. It’s truly a game-changer in the world of music production and an exciting glimpse into the future of AI innovation.
One of the most notable generative AI interfaces is StyleGAN, which is an AI-driven algorithm that can generate high-quality images of people that don’t exist in reality. StyleGAN is a type of generative adversarial network (GAN) that is trained on large datasets of images to generate new, high-quality images that don’t actually exist in the real world.
The key innovation of StyleGAN is that it can generate images with much greater control over various attributes, such as facial features or clothing styles, making it possible to create highly realistic images of people that don’t actually exist.
At a broad level, StyleGAN operates by training two distinct neural networks simultaneously. The initial network, referred to as the generator, receives input in the form of a random noise vector and produces an image that aims to replicate the style and characteristics present in the training data.
Concurrently, the second network, known as the discriminator, is trained to differentiate between real images and the images generated by the generator. As training progresses, the generator learns to generate increasingly realistic images that deceive the discriminator. Eventually, the generator becomes adept at producing images that are incredibly similar to genuine photographs, making them exceedingly difficult to distinguish.
While the previously discussed generative AI tools are widely recognised, there are several other notable tools in the spotlight worth mentioning. Generative AI encompasses various modalities, including text, imagery, music, code, and voices. Below are some popular AI content generators across different domains.
As you can see, there is pretty much an AI tool out there for every possible need. These tools provide exciting possibilities in their respective domains and can contribute to creative content generation, enhanced coding experiences, and advanced voice synthesis capabilities.
It’s safe to say generative AI is a powerful tool for creating new content and can potentially revolutionise many industries. It relies on deep learning models, particularly language models, which are trained on large amounts of text data and can generate new text based on the patterns they have learned.
While generative AI has enormous potential, it raises important ethical and social questions that must be addressed. As technology continues to develop, it is up to us to use it responsibly and ensure that it benefits society as a whole.
With its incredible capabilities and potential for revolutionising entire industries, generative AI promises to be one of the most dynamic technologies of this century – but only if we use it responsibly.
If you’re looking for a partner that can help you implement cutting-edge technologies in an effective way, look no further – we are here to help. We believe in using data-driven decision-making and modern tools like generative AI to enhance productivity and end results. Book a discovery call to discuss how we can help you use technology to level up and stand out from the crowd.
May 19, 2023